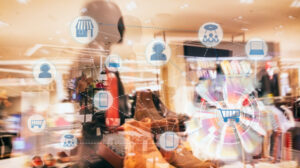
At every step of operations, from queue management and inventory management, to brand building and recall, retail analytics is a must for brick-and-mortar stores today. We look at the advantages of retail analytics and the 4 different types of retail analytics that retailers have to track.
To understand retail analytics better, let’s first dive into a real-life example of how a global jewellery retailer saw increased revenues and gained a deeper understanding of consumer behaviour patterns using retail analytics tools.
Headquartered in Bermuda, Signet Jewellers is an international retailer of diamond jewellery. The company has 3,000 brick and mortar stores in the US, the UK and Canada. Just as any other brand does today, Signet Jewellers was pulling all stops to understand consumer buying patterns, stay relevant to eCommerce trends, and maximise sales in physical stores.
But, while the company had reams of consumer data available in its systems, it still struggled to consolidate all data and draw contextual insights on various aspects of the business. This was primarily because data was available in silos across departments, and each department had employed its own data management vendor to tackle the data. This meant a burden on the IT team to get familiar with each vendor’s data solution, high operational and maintenance costs, and reactive business planning, rather than proactive business forecasting.
To find a solution to these challenges, Signet Jewellers partnered with Tableau. Through the partnership, it;
- It enabled the IT team to consolidate and centralise data into a single enterprise data warehouse (EDW)
It created dashboards starting with one unit of the business – the real estate division. The dashboards, for example, gave quicker insights from data and made lease negotiations more agile and flexible. Similarly, it also drew revenue forecasts and forecasts on SKU requirements based on consumer preferences and purchase behaviour. - It lowered IT overhead costs
- It increased revenues by analysing millions of transaction data and identifying opportunities that the brand can capitalise on
The result? With Tableau, Signet Jewellers saved over USD 250K by enabling teams to find data patterns through a single maintenance dashboard. It analysed over 75 million PoS transactions and identified opportunities worth USD 10 million and more.
Signet Jewellers case study is a classic example of the impact that analytics-based decision making (or retail analytics) can have on retail businesses.
Retail analytics is the practice of gathering and analysing data related to supply chain, procurement, sales and consumer demand to make better business decisions. According to research, the retail analytics market is witnessing such demand that it is set to grow from USD 5.84 billion in 2021 to USD 18.33 billion by 2028.
Advantages of Retail Analytics
In today’s times, retail analytics is crucial because;
- We live in times when retailers need to market and engage with their customers across multiple channels like in-store campaigns, social media initiatives, web initiatives and more. In each channel, there’s potential to collect tons of data that can throw light into what a customer wants, and what you need to do as a brand, to stay ahead of the market.
A Merit expert adds, “Of course, it works the other way around too. Even though you have a chain of brick-and-mortar stores, your customers are so connected online that they are looking you (and your competitors) up online before visiting your store. They check product availability, compare prices, read reviews and then only take the decision to come in-store. The point is – you need to plan your visibility strategy based on what your customers want. For this, customer data and intelligence from past customer experiences are critical.”
According to data, 81% of retail shoppers begin their shopping journey by researching a brand online. Another data suggests that 59% of shoppers who visit in-store use their mobile devices to find out about deals, coupons and price of the products they want at competitor stores.
- It can help you take quicker, more proactive business decisions related to managing procurement, inventory and supply chain, and meeting customer demands.
Did you know that poor inventory management costs retailers nearly USD 300 billion each year?
- It can help you run more targeted, personalised online and offline campaigns which can result in higher sales and greater customer loyalty
Retailers who have personalised their campaigns have recorded a USD 20 higher return per every dollar they have invested
- It can optimise your in-store and ecommerce operations. For example, it can throw insights into how you need to design the layout for your store, which products you need to stock where, how you need to improve your service quality and such.
Did you know? Retail analytics has gone to such depth that it can help retailers manage store queues better (so that customers don’t leave without checking out due to long wait times). There is also software that can track which aisles the customer is visiting and where they are spending the most time, so that retailers can use this data to stock high-demand products in these shelves.
Types of Retail Analytics
While the nuances of analytics can vary greatly from store to store and region to region, retailers can rely on these four analytics to get the most out of their customer data.
Descriptive Analytics shows insights into what has happened after the event has occurred. For example, retailers can use descriptive analytics to analyse how an in-store seasonal sale has performed, or how a social media campaign has performed. Usually, retailers use this method to review the year that has passed, to draw inferences about what can be done better going forward.
Diagnostic Analytics, as the name suggests, analyses why something happened. For example, it can show insights into why a marketing campaign did not get the estimated conversions or engagement, or why a particular product did not sell as anticipated.
Predictive Analytics uses past data on customer browsing and purchasing behaviour to predict future forecasts. For example, retailers can use predictive analytics to bundle products together, stock specific products during peak season times, or offer discounts or coupons on some products to increase repeat purchases and loyalty. Retailers can also use predictive analytics to plan their inventory and supply chain, and arrive at the right pricing strategy based on past demand for the product/s.
Prescriptive Analytics uses AI and machine learning programs to prescribe what retailers can do next with the available insights into the various aspects of their business. For example, during Christmas, if the store saw a higher footfall and billing than anticipated, and the process from selection to purchase took longer because the store was understaffed, prescriptive analytics suggest the ideal staff to customer ratio to ensure a smoother customer experience and quicker checkout times.
Today, thanks to retail analytics, brick and mortar retailers are on par with ecommerce retailers in terms of providing personalised customer experiences, and meeting customer needs. What remains to be seen is how far AI and ML can go in optimising retail operations and making the customer journey as seamless as it is in the ecommerce world.
Merit’s Expertise in Retail Data and Intelligence
Our state-of-the-art retail data harvesting engine collects raw data and provides actionable insights
- Three to four times faster than standard scrapers
- At lower cost
- With Increased accuracy (up to 30% compared to standard scrapers)
Our powerful, new scraper engine can gather massive data sets from multiple sites and geographies in real-time so you can stay informed on customer behaviours and market trends.
Merit’s eCommerce and retail data engine provides a high degree of confidence in insights generated from analytics – thanks to confidence in the data quality and access to enriched data.
To know more, visit: https://www.meritdata-tech.com/service/data/retail-data/
Related Case Studies
-
01 /
AI Driven Fashion Product Image Processing at Scale
Learn how a global consumer and design trends forecasting authority collects fashion data daily and transforms it to provide meaningful insight into breaking and long-term trends.
-
02 /
Automated eCommerce Data Harvesting at Scale and Speed
This company reduced their cost by 15% & automated 80% of their quality assurance & data integrity checks.