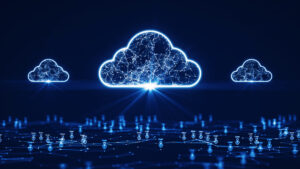
At the risk of repeating a cliché, let us say it once more: Enterprises across the world are dealing with extremely high-volumes of data. This data explosion is primarily being led by the digital transformation of business processes across the company.
In addition to operational data residing in the ERP, CRM and other business systems, companies have also ended up with a plethora of SaaS-based tools, which are being used by different departments and functions. For instance, the marketing department today relies heavily on various digital tools for social media management, media monitoring, marketing data management, customer success management, customer support, spend analytics, etc. Each of these tools has some embedded analytics, dashboards and insights, but that’s not enough for overall marketing decision-making.
The need of the hour is to unify and integrate data from a wide range of business applications, databases, data warehouses and data lakes and bring it to a central destination for Business Intelligence (BI) and analytics. To garner holistic insights and intelligence, the only way forward is to have a Cloud BI strategy in place.
Here, we highlight some of the emerging technologies that are making it easier to migrate analytics workloads to the cloud and get ready for a world that’ll be powered by AI and advanced analytics.
Trend #1: Distributed Data Lake Architecture Spanning Multiple Clouds and Data Sources
Advanced analytics revolves around the ability to ingest data from both structured and unstructured data. On one hand, there’s structured data available in business systems like the ERP and SaaS tools. These are mostly quantitative data points and it’s comparatively a simple process of unifying data from multiple sources.
On the other hand, there’s also unstructured data from reviews on e-Commerce platforms, social media comments, pdf files, documents, legal sources, etc. To leverage data sets from these types of sources, we use a data lake architecture.
A data lake is a central repository that stores structured, semi-structured and unstructured data. By using technologies like metatags and semantic layers, it is possible to retrieve relevant data points from unstructured sources in a data lake.
Merit’s Senior Solutions Architect adds, “Today, platforms like GCP offer solutions like BigLake, making it possible to unify data lakes and warehouses spanning multiple clouds. It is designed to take care of security and data governance requirements, while also providing data access as needed.”
Trend #2: Usage of AI, ML and NLP in Analytics
Now that we have the ability to process unstructured data at scale, modern analytics tools are able to leverage technologies like Natural Language Processing (NLP), Computer Vision for image processing, and Machine Learning, to automate the process of data analytics.
By building the right AI model, data analytics teams are able to reduce time taken from “data-to-insight”.
Using AI, data scientists can do the following:
- Similarity Identification: Spot data trends based on similar data points that were previously analysed
- Next Best Recommendations: Offer recommendations on what action must be taken based on insight gathered
- Predictive Modelling: Make predictions on what may happen
- Prioritisation and classification: Automatically prioritise and help business users decide which insights to act on. For instance, if a particular risk has been identified, the associated risk mitigation action item is pushed up the prioritisation queue
- Image processing: Using machine learning and computer vision techniques, it is now possible to identify images and use data points from these images to make decisions
Trend #3: Cloud Data Loss Prevention (DLP), Data Masking and Other Compliance Requirements
One of the most aspects of running analytics on the cloud is to ensure there is no data loss. It is also paramount that data integrity is maintained, and all data privacy, security and governance requirements are followed.
Google’s BigLake tables, for instance, allows data scientists to design data masking rules to ensure sensitive data is protected. All user query requests are audit logged, so it becomes easy when compliance audits are conducted.
Trend #4: Unified Governance and Data Management using a single tool
When it comes to managing big data workloads, the process of cloud governance becomes complex. Today, data science teams are looking for an easier way to “centrally discover, manage, monitor, and govern their data across data lakes, data warehouses, and data marts”.
In the GCP environment, for instance, there’s a solution called Dataplex that make it possible to do that. Through this single tool, GCP users can:
- Centrally manage data security and governance
- Ensure data quality and protect data lineage
- Unified metadata management
- Easily discover and search for new data
The equivalent is possible in other cloud environments like AWS and Azure.
In conclusion, the world of cloud computing for big data is evolving at breathtaking speed. The above four trends we’ve highlighted in this blog has certainly reduced the complexity for data engineering and data science teams.
Technologies like AI and NLP are now more accessible than ever, thanks to in-built capabilities in platforms like Azure AI and Google Cloud AI. Additionally, the challenges associated with high-volume data management are also reducing, thanks to modern tools like Dataplex.
Having said that, it is now more important than ever to choose the right partner for your Big Data and Analytics management requirements. You will need an experienced technology partner that has the ability to handle massively large-scale datasets, with proven governance and compliance capabilities.
Merit’s Expertise in Cloud Migration and Analytics on the Cloud
Merit works with a broad range of clients and industry sectors, designing and building bespoke applications and data platforms combining software engineering, AI/ML, and data analytics.
We migrate legacy systems with re-architecture and by refactoring them to contemporary technologies on modern cloud ecosystems. Our software engineers build resilient and scalable solutions with cloud services ranging from simple internal software systems to large-scale enterprise applications.
Our agile approach drives every stage of the customer journey; from planning to design development and implementation, delivering impactful and cost-effective digital and data transformations.
To know more, visit: https://www.meritdata-tech.com/service/code/digital-engineering-solutions/
Related Case Studies
-
01 /
A Unified Data Management Platform for Processing Sports Deals
A global intelligence service provider was facing challenge with lack of a centralised data management system which led to duplication of data, increased effort and the risk of manual errors.
-
02 /
A Hybrid Solution for Automotive Data Processing at Scale
Automotive products needed millions of price points and specification details to be tracked for a large range of vehicles.