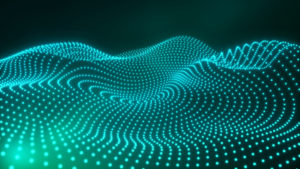
In one of our earlier blogs, we wrote about how Accenture used the Google Cloud Platform (GCP) to power its business intelligence transformation initiatives. The global management consulting firm leveraged the capabilities of the Google Data Cloud to modernise its overall data stack, not only to power its business intelligence (BI) efforts but also to drive the implementation of artificial intelligence and machine learning (AI/ML) solutions.
In this blog, we highlight one key aspect of Accenture’s move to GCP – the usage of Google Data Lake to store and process unstructured and semi structured data as well.
The role of Google Data Lake
It is critical for decision-makers to understand that a data lake is fairly different from a data warehouse. A data warehouse is a storage solution to store high volumes of data but it is well-suited for regular reporting and analytics. For instance, if you’re looking for BI around monthly sales volumes, marketing campaign spends on a month-on-month basis, etc., a data warehouse works very well. In other words, a data warehouse is ideal for repeatable analytics.
But, what if you want to set up new types of advanced analytics experiments? You may want to leverage AI/ML techniques to make sense of the high volume of incoming raw data. With Google Data Lake, it is fairly seamless to ingest massive amounts of raw data via batch or stream processing, without having to transform it. You can transform data as and when you need to use it for analytical modeling, but otherwise, it can remain in the data lake.
Google’s Data Lake is well-suited to store and process large amounts of full-fidelity data – of varying types from various data sources. This could be from on-premise systems, edge computing devices, other cloud data warehouses, SaaS applications, etc.
A data science expert at Merit says, “One reason why companies are moving from Apache Hadoop or Spark workloads to Google Data Lake could revolve around Total Cost of Ownership (TCO). But, keep in mind that the time spent by data engineers in dealing with infrastructure bottlenecks is also an important factor to consider. If you’re already on the GCP platform, it makes sense to explore Google Data Cloud.”
Key Advantages of implementing Google’s Data Lake
Lower TCO (Total Cost of Ownership): One of the biggest challenges when it comes to on-premise data lake implementations is cost. This is especially true for industry intelligence companies that take in data from a whole variety of sources including websites, e-commerce platforms, pdf documents, photos, etc. From a cost perspective, it makes sense to re-host your data lake on GCP, completely eliminating your on-premise data lake
Data Processing Made Easy: This is one of the biggest advantages of a Google Data Lake. Often, analytical processing is resource-intensive and slow. By using Google’s infrastructure, it is possible to auto scale compute resources for analytics processing, without provisioning new hardware.
Don’t let your Data Lake become a Swamp: Often, companies end up implementing a data lake architecture that becomes difficult to scale. Using a cloud-native Data Lake like Google’s, it makes life easier for data scientists, data engineers, and developers who don’t want to get caught up in dealing with infrastructure and scaling bottlenecks.
Why Pandora moved 7 petabytes of data to Google Data Lake
Pandora, the leading music and podcast delivery platform, moved over 7 PB of data to Google Data Lake. They did that for several reasons but some of the biggest reasons were – easier in terms of implementation, major cost savings, the ability to integrate with other solutions and using Google BigQuery to run SQL queries on the data lake data, at scale.
Companies are also migrating Apache Hadoop and Spark workloads to Google Data Lake, and this process is made easy by using Dataproc. Additionally, GCP’s capabilities in the areas of data management, security, and governance are well-proven.
Merit Group’s expertise in Data Lakes
Merit Group partners with some of the world’s leading B2B intelligence companies within the publishing, automotive, healthcare, and retail industries. Our data and engineering teams work closely with our clients to build data products and business intelligence tools that optimise business for growth.
The first step to getting BI right is to make sure your data storage strategy is optimal – and this may require advice on the right type of data lake to be implemented. Merit’s team of data engineers will work closely with your CIO and other key decision makers to lay the foundation for a robust data intelligence and BI roadmap that is future-proof, including providing unbiased knowledge sharing on all the technologies/tool options available.
If you’d like to learn more about our service offerings or speak to a data science expert, please contact us here: https://www.meritdata-tech.com/contact-us
Related Case Studies
-
01 /
Automotive Data Aggregation Using Cutting Edge Tech Tools
An award-winning automotive client whose product allows the valuation of vehicles anywhere in the world and tracks millions of price points and specification details across a large range of vehicles.
-
02 /
Formularies Data Aggregation Using Machine Learning
A leading provider of data, insight and intelligence across the UK healthcare community owns a range of brands that caters to the pharmaceutical sector and healthcare professionals in the UK.