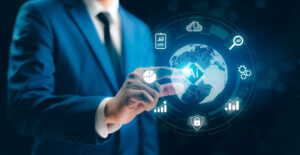
In the digital age, data is the cornerstone of business organisations. Especially with the exponential growth of data (the global data sphere is expected to grow to 175 zettabytes by 2025), businesses need to establish effective data management practices to make informed decisions, enhance operational efficiency, and maintain competitive advantage.
In recent years, artificial Intelligence (AI) and machine learning (ML) have started revolutionising data management by automating processes, improving data accuracy, and providing deeper insights. These technologies enable organisations to handle vast amounts of data more efficiently, uncover hidden patterns, and predict future trends.
In this article, we focus on 5 innovative ways to integrate AI and ML with data management, offering practical strategies to harness the full potential of these technologies for enhanced data handling and analysis.
Role of AI & ML in Enhancing Data Quality & Accuracy
We saw that AI & ML are revolutionising data management. But how are they doing it? Let’s delve deeper into this.
AI and ML technologies are transforming data management by automating data cleansing and validation processes. These technologies can identify and correct errors, inconsistencies, and duplicates in large datasets, ensuring higher data quality and reliability. Machine learning algorithms can learn from historical data to predict and rectify anomalies, while AI-driven tools can continuously monitor data streams for real-time validation.
For instance, AI can automate the detection of outliers and missing values, which are common issues in large datasets. Machine learning models can be trained to recognise patterns and relationships within the data, enabling them to fill in missing information accurately. Additionally, natural language processing (NLP) techniques can be used to standardise and normalise data from various sources, ensuring consistency across the dataset.
Let’s look at real-life examples of how these technologies are impacting data management across sectors.
In healthcare, AI & ML are used to clean and validate patient records. For example, IBM Watson Health employs machine learning algorithms to identify and correct errors in medical records, ensuring accurate patient information and improving treatment outcomes.
In financial institutions, AI-driven tools are used to cleanse and validate transaction data. JPMorgan Chase, for instance, utilises machine learning models to detect fraudulent transactions and ensure the accuracy of financial records, enhancing both security and data quality.
Furthermore, retail companies like Amazon use AI to manage product data, whereas machine learning algorithms help in identifying and correcting discrepancies in product descriptions, prices, and inventory levels, ensuring accurate and up-to-date information for customers.
Global Perspective with a Focus on EU Regulations
The General Data Protection Regulation (GDPR) in the European Union has set high standards for data quality and accuracy. Under GDPR, organisations are required to ensure that personal data is accurate and up-to-date. AI and ML can play a crucial role in meeting these requirements by automating data quality checks and validations.
For example, GDPR mandates that individuals have the right to rectify inaccurate personal data. AI-driven data management systems can facilitate this by automatically identifying and correcting inaccuracies, thus ensuring compliance with GDPR. Additionally, AI tools can help organisations maintain data accuracy by continuously monitoring and updating records, reducing the risk of non-compliance.
Globally, the emphasis on data quality is growing, with regulations similar to GDPR being adopted in various regions. AI and ML technologies provide a scalable and efficient solution to meet these regulatory requirements, ensuring high data quality standards across industries.
Role of AI & ML in Data Integration
Artificial Intelligence (AI) and Machine Learning (ML) are transforming how organisations manage data integration from various sources by automating the extraction, transformation, and loading (ETL) processes, thus significantly reducing the need for manual work. By using AI and ML, organisations can easily combine data from different places, ensuring that it is consistent and accurate.
One major benefit of these technologies is the reduction of manual effort. AI and ML algorithms can automatically identify, classify, and map data from different sources, which cuts down on manual data entry and minimises human error. This automation allows data professionals to focus on more strategic tasks instead of routine data processing.
Additionally, AI and ML make data integration faster and more efficient. They can handle large amounts of data in real-time, keeping information up-to-date and readily available for analysis. This speed leads to quicker decision-making and a more agile response to business needs.
Role of AI & ML in Advanced Data Analytics & Insights
AI & ML enhance data analytics through advanced methods like predictive analytics and anomaly detection.
Predictive analytics uses historical data to forecast future outcomes. For example, retailers apply machine learning algorithms to predict inventory needs, helping them optimise their supply chains and avoid stock shortages. Similarly, businesses can forecast customer behaviours and potential risks, allowing them to plan more effectively.
Anomaly detection is another key technique that AI and ML employ to spot unusual patterns or outliers in data. This method is crucial for identifying potential fraud or system failures. For instance, ING Group, a Dutch multinational banking corporation, uses AI and ML to monitor transactions in real-time. This allows them to quickly detect and respond to suspicious activities, enhancing the security of customer accounts.
Companies like SAP also leverage these advanced analytics techniques. SAP provides predictive analytics tools that help businesses across Europe forecast market trends, optimise operations, and improve customer experiences. By integrating AI and ML into their analytics strategies, organisations can significantly enhance their ability to make informed decisions and respond to challenges more effectively.
Role of AI & ML in Improving Data Security & Compliance
AI & ML can analyse vast amounts of data in real-time, detecting anomalies and potential security breaches that might go unnoticed by traditional methods. Their algorithms can identify patterns and trends in user behaviour, facilitating early detection of security threats and enabling proactive measures to mitigate risks.
For instance, AI-driven threat detection systems can automatically sift through extensive datasets to uncover hidden risks, enhancing overall security measures. These systems can also adapt to new threats by learning from past incidents, making them highly effective in combating evolving cyber threats such as polymorphic malware and zero-day exploits.
Siemens, for instance, employs AI/ML for anomaly detection in its cybersecurity framework. By continuously monitoring network traffic and system behaviour, Siemens can identify and respond to potential threats in real-time, ensuring the security of its operations. Similarly, SAP leverages AI/ML to provide advanced security solutions for its clients. These solutions include predictive analytics for threat detection and automated compliance checks to ensure adherence to GDPR and other data protection regulations.
Role of AI & ML in Streamlining Data Governance & Management
These technologies help organisations manage data more effectively by ensuring data quality, integrity, and compliance with regulatory standards. AI/ML can automate various aspects of data governance, making it easier to maintain accurate and reliable data across the organisation.
One significant area where AI and ML contribute is data cataloguing. They can automate the creation and maintenance of data catalogues, which are essential for organising data assets. By using machine learning algorithms, organisations can automatically discover, classify, and index data from different sources. This automation reduces the manual effort needed and keeps data catalogues up-to-date, making it easier to find and use relevant data.
AI and ML also enhance metadata management, which provides crucial information about data, such as its origin and usage. These technologies can automatically generate and update metadata, ensuring it accurately reflects the current state of the data. This capability helps organisations maintain data consistency and improves the efficiency of data-related processes.
Additionally, AI and ML can automate the enforcement of data governance policies. By continuously monitoring data usage and access patterns, AI-driven systems can detect and respond to policy violations in real-time. This proactive approach ensures compliance with data protection regulations and minimises the risk of data breaches.
In conclusion, AI and ML are transforming how organisations manage data. By improving data quality, automating integration and processing, and offering advanced analytics, these technologies drive efficiency and innovation. They also enhance data security and compliance while streamlining governance. As European organisations and others worldwide adopt AI and ML, they become better equipped to meet regulatory standards and strengthen their data strategies. Embracing these technologies is vital for staying competitive in the digital age.
Merit Quote: “The integration of AI and ML into data management not only streamlines processes but also accelerates real-time data analysis, allowing organisations to respond nimbly to market demands and emerging trends.”
Merit’s Expertise in Data Aggregation & Harvesting Using AI/ML Tools
Merit’s proprietary AI/ML tools and data collection platforms meticulously gather information from thousands of diverse sources to generate valuable datasets. These datasets undergo meticulous augmentation and enrichment by our skilled data engineers to ensure accuracy, consistency, and structure. Our data solutions cater to a wide array of industries, including healthcare, retail, finance, and construction, allowing us to effectively meet the unique requirements of clients across various sectors.
Our suite of data services covers various areas: Marketing Data expands audience reach using compliant, ethical data; Retail Data provides fast access to large e-commerce datasets with unmatched scalability; Industry Data Intelligence offers tailored business insights for a competitive edge; News Media Monitoring delivers curated news for actionable insights; Compliance Data tracks global sources for regulatory updates; and Document Data streamlines web document collection and data extraction for efficient processing.
Key Takeaways
Importance of Data: In the digital age, effective data management is crucial for informed decision-making and maintaining a competitive edge.
Role of AI and ML: These technologies automate data management processes, enhance data accuracy, and provide deeper insights.
Data Quality and Accuracy: AI and ML improve data quality by automating cleansing and validation, identifying errors, and ensuring reliable datasets.
Data Integration: AI and ML streamline the extraction, transformation, and loading (ETL) processes, significantly reducing manual effort and improving efficiency.
Advanced Analytics: Techniques like predictive analytics and anomaly detection help organisations forecast trends and identify unusual patterns, enhancing decision-making.
Data Security and Compliance: AI and ML enhance data security by detecting anomalies in real-time and ensuring compliance with regulations like GDPR.
Streamlining Governance: These technologies automate data cataloguing and metadata management, making it easier to maintain data integrity and comply with regulations.
Global Relevance: The adoption of AI and ML for data management is growing worldwide, with a focus on meeting regulatory standards and enhancing data strategies.
Competitive Advantage: Embracing AI and ML is essential for organisations to stay competitive in an increasingly data-driven landscape.
Related Case Studies
-
01 /
AI Driven Fashion Product Image Processing at Scale
Learn how a global consumer and design trends forecasting authority collects fashion data daily and transforms it to provide meaningful insight into breaking and long-term trends.
-
02 /
Advanced ETL Solutions for Accurate Analytics and Business Insights
This solutions enhanced source-target mapping with ETL while reducing cost by 20% in a single data warehouse environment