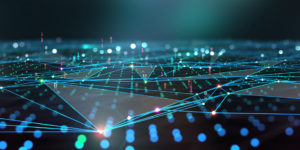
“High-quality data is essential for policy and decision making and underpins your organisation’s strategic outcomes,” writes the UK Government Data Quality Hub. “Poor quality data, including data that is inaccurate, incomplete, or out of date, is data that is not fit for purpose.”
Not only is inaccurate, incomplete, or out-of-date data “not fit for purpose”, it could hamper effective data-driven decision-making and actively work against an organisation’s best interests. Organisations are increasingly using business intelligence (BI) to determine both immediate and long-term strategies. By combining data from reputable sources, they can make more intelligent, better-informed decisions in a timely manner, and gain a competitive edge over their competition.
But the Government’s Data Quality Hub has overlooked one further determinant of poor-quality data: metrics that are inaccessible, or difficult to employ.
Business intelligence is changing
Business intelligence is not new: it has just become more accessible. The term was coined in the 1960s, says Tableau, and “developed in the 1980s alongside computer models for decision-making and turning data into insights before becoming a specific offering from BI teams with IT-reliant service solutions”.
Those IT-reliant solutions may have been acceptable at a time when computers were mere adjuncts to business, but not today. BI tools, like Microsoft Power BI and tools within Excel, are accessible across the organisation. Moreover, staff are increasingly presented with a range of data sources, and tasked with performing their own self-service BI operations, rather than waiting for a central IT service team to deliver that intelligence on their behalf.
The growth and growth of data
The market for self-serve BI is projected to grow by 15.5% (CAGR) by 2026 as organisations reassign this business function from specialists, who could be expected to keep abreast of standards and conventions, to those whose responsibilities lay elsewhere, in driving efficiencies, increasing sales, or implementing social change.
Further, the range of data is wider than ever before – and continuing to grow, with global data expected to increase by 23% CAGR by 2025. Alongside first-party data, like sales and profit, enterprises increasingly make use of both second-party and third-party metrics to derive BI. It is likely that these data will be stored on diverse public and private cloud, and on-premise locations, and any expectation that staff know where that data can be found would add another level of complexity.
Simplification through data virtualisation
This requirement can be mitigated with data virtualisation, which tracks available data sources and presents them as though they were a single resource. The data itself is not shifted from its original locations, but bridges between the systems used by those performing the BI function, and the servers on which the data is stored, makes the distinction between local and remote irrelevant.
More importantly, using data virtualisation, obviates the need to duplicate the diverse data sets in a specific location. This reduces the likelihood of errors being introduced at the extract, transform, load (ETL) stage, for a more accurate and dependable result.
Deriving accurate insight through visualisation
It is frequently true that a single source of data can only tell part of a story, and that multiple data sources must be combined to provide truly valuable insight. For example, an organisation may compile its own sales data using returns from across its outlets and could use this to judge which are under performing, with a view to closing unprofitable venues.
While this process may highlight which outlets are struggling, it is unlikely to give context to the result. Therefore, correlating that data with additional metrics, like weather conditions, traffic problems, or infrastructure work, may explain – and excuse – the under performance of each outlet marked for closure. Indeed, accounting for these conditions may even highlight that some more profitable outlets are the poorest performers within the organisation.
Analysis of this kind would be difficult and time consuming without data visualisation. Retrieving the relevant data sets, checking that they are current, extracting the necessary metrics for each location, and using them to produce accurate and meaningful comparisons would be a considerable workload.
It would also require navigation of several incompatible formats and may call on the services of an increased workforce. In this instance, therefore, data visualisation has the potential to deliver savings, even before real-world cost efficiencies are implemented.
Reducing time to output
Further, because virtualisation leaves the data where it already sits, it can start to deliver insight more quickly. Contrast this with an ETL-focused workflow, where it may be necessary to devise an appropriate infrastructure for the project, build it, then import the data.
All of this must happen before users can start working with data that can only ever present a snapshot of conditions at a specified moment. As the original data sets age at different rates, their variance from the copy will shift in an unpredictable manner, and any points of comparison are almost certain to drift. This makes any insight derived from the data less reliable, and that reliability continues to decrease over time.
With data virtualisation, on the other hand, there is little or no need for capital expenditure, no requirement for infrastructure design and fit-out, and no expectation that business users familiarise themselves with new structures or processes. The time required to deliver a return is reduced – and so are costs.
Additional benefits of data virtualisation
As data remains in place, costs can be reduced as workloads are lighter. This is particularly true when renting cloud assets by time, capacity, or throughput. When changes are made to one or more of the master data sets, they are immediately visible to the BI platform, since there is no need to transfer it, manually or at timed intervals, to client systems. Thus, as users run new queries, they will always return a relevant, up to date result, for a reliable single version of truth.
However, when data is virtualised, users will frequently find themselves accessing data lakes, marts, warehouses and more, which have been established at various times, using a variety of underlying technology, and conforming to the best practices of a range of unknown teams. There is no reason there should be correlation between any of them.
This has the potential to counterbalance one of the major benefits of virtualisation: its ability to free users from any knowledge of the underlying structure. Thus, a semantic layer should be deployed to disguise as fully as possible any differences between the various assets.
Applying a semantic layer
A semantic layer makes data more accessible to its human users, by correlating data points with common business terms, like customer, revenue, and return. These terms are themselves stored alongside the data and swapped in where structural language might otherwise be used. This reduces complexity and allows a greater range of stakeholders to use the data themselves. This, in turn, helps maximize its utility, the value that can be extracted from it, and the return it can deliver.
“A semantic layer provides the enterprise with the flexibility to capture, store, and represent simple business terms and context as a layer sitting above complex data,” writes Lulit Tesfaye. “A semantic layer is not a single platform or application, but rather the realisation or actualisation of a semantic approach to solving business problems by managing data in a manner that is optimised for capturing business meaning and designing it for end user experience.”
The idea of a semantic layer, like BI, is not new. It was patented in 1991, and today increases the power of BI tools by making them immediately comprehensible and accessible, since just as users do not need to know or understand where their data is stored, the semantic layer means they do not need any knowledge of the structure or formatting of the data itself. Neither should they need to learn complex query languages to extract the answers they need.
More importantly, with a semantic layer between the user and the data, they can work with multiple data sources as though they were a single pool, even if those sources do not share a common structure. This is logical. Why should the user need to understand somebody else’s organisational process before they can do their own job?
Semantic layer and AI/ML
As enterprises increasingly adopt AI/ML to accelerate their intelligence and decision-making processes, the semantic layer will become even more important. Just as it has long allowed BI users to derive insight from data without needing to understand how that data is structured, it now also allows AI to convert the kind of language routinely used by the humans with whom they interact into complex queries. This will allow them to deliver more meaningful insight more quickly.
Making data more accessible through virtualisation and simplifying the process of using it to deliver insight, courtesy of a semantic layer, will help organisations to extract maximum value from the metrics they hold – and, even, the data they frequently overlook.
Almost three quarters of all data is never used for analytics, and data is frequently gathered, stored, and left to stagnate. This represents an enormous lost opportunity – but it is one that could be recouped now when staff are able to self-service their ongoing BI requirements.
Related Case Studies
-
01 /
A Hybrid Solution for Automotive Data Processing at Scale
Automotive products needed millions of price points and specification details to be tracked for a large range of vehicles.
-
02 /
Advanced ETL Solutions for Accurate Analytics and Business Insights
This solutions enhanced source-target mapping with ETL while reducing cost by 20% in a single data warehouse environment