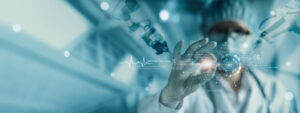
In earlier blogs, we looked at the various facets of AI applications in global healthcare. We saw how AI can bring structured and unstructured data together, how it can aid in healthcare data harvesting, and how it can contribute to data validation.
In the last few years, despite regulatory hurdles and extensive data protection mechanisms (which are a necessity in this industry), AI has penetrated deep into the healthcare market.
A Merit expert adds, “Today, we can find AI applications on the administrative side, where more efficiency is being brought into patient management, insurance claim management, and clinical patient data management. It is being applied in the public health space, where public health records, including scans and reports, are being analysed to identify patterns in diseases and illnesses for designing preventive care or minimising risk.”
AI’s Greatest Areas of Impact in Healthcare
We can see its application in medical research and medical training, in clinical trials and drug testing, and in teaching medical students effective ways to treat illness or health complications.
A 2023 study on AI applications in healthcare reveals that its impact has been the highest on healthcare analytics (23%), medical diagnostics (22%) and telehealth (19%). Other areas where the application has begun but is still in its infancy are medical robots, hospital management, clinical decision support and clinical trials, public health management, cybersecurity and personalised healthcare.
AI is not without its risks
Despite the giant strides it has made in improving diagnostic accuracy, enabling early detection of diseases, enabling development of personalised treatment plans and the like, the technology also comes with its fair share of risks. The foremost being, privacy and security risks due to large-scale data collection and ethical challenges surrounding AI’s decision-making capabilities.
Let’s look at the risks of AI in healthcare in more detail.
Data privacy and security
AI relies on vast amounts of sensitive patient data, making it susceptible to breaches, hacking, and unauthorised access. Protecting patient privacy and ensuring secure data storage and transmission are crucial.
Bias and fairness
AI algorithms can inherit biases from the data they are trained on, leading to discriminatory outcomes. These biases can result in unequal access to healthcare, misdiagnoses, and unequal treatment for certain populations. Efforts are needed to mitigate bias and ensure fairness in AI systems.
Lack of transparency and interpretability
Some AI algorithms, such as deep learning models, are considered “black boxes” as they provide results without clear explanations. This lack of transparency raises concerns about accountability, trust, and the ability to understand and validate the reasoning behind AI-generated recommendations or decisions.
Overreliance and errors
Relying too heavily on AI systems without proper validation or human oversight can lead to errors or incorrect diagnoses. AI should be viewed as a supportive tool rather than a replacement for healthcare professionals.
Ethical dilemmas
AI raises complex ethical questions, such as determining responsibility in cases of AI-generated decisions, ensuring informed consent for AI-driven treatments, and addressing the potential loss of the human touch in patient care.
Potential Solutions to Stem Risks from AI Applications
While AI in healthcare presents risks, there is hope in finding solutions to mitigate them. Here are some potential solutions we can explore.
Anonymity and encryption to protect and secure
Ensure strict encryption, anonymisation, and access controls to protect patient data from breaches and unauthorised access.
Homomorphic Encryption
For example, one way in which hospitals can protect their data is through homomorphic encryption. This is an advanced cryptographic technique that allows computation to be performed on encrypted data without decrypting it. This means that even if the data is intercepted or accessed without authorisation, it remains encrypted and unintelligible to unauthorised parties.
With homomorphic encryption, the hospital can securely store patient data in a cloud-based database or transmit it to other healthcare providers or researchers. The data remains encrypted throughout, minimising the risk of unauthorised access or data breaches. It can also be applied to protect patient privacy. For example, personal identifying information, such as names, addresses, and social security numbers, can be removed or replaced with pseudonyms, making it challenging to link the data back to specific individuals.
Role based access control
Lastly, with homomorphic encryption, role-based access control (RBAC) systems can be implemented, where individuals are granted access based on their roles and responsibilities. This restricts access to sensitive patient information only to authorised healthcare professionals who need it for diagnosis, treatment, or research purposes.
Treating Bias with Diversity in Datasets
Develop diverse and representative training datasets, employ bias detection algorithms, and implement fairness metrics to minimise bias and ensure equitable healthcare outcomes.
For example, let’s say a hospital is using AI to predict the likelihood of readmission of patients with heart failure. It can train the AI system to use historical patient data including demographic information, medical history and treatment outcomes to measure its outcomes fairly, accurately and transparently, and identify bias or flaws in the system.
Bias Detection Algorithms
Bias detection algorithms can analyse an AI system’s predictions and identify any potential biases in the results. For instance, the algorithm might flag instances where the AI system consistently predicts a higher readmission rate for certain demographic groups, indicating the presence of bias.
To ensure equitable healthcare outcomes, the hospital can implement fairness metrics which can assess the performance of the AI system across different demographic groups, monitoring for any disparities or unfair treatment.
If the metrics reveal discrepancies, the hospital can take corrective actions, such as recalibrating the AI model or adjusting decision thresholds to ensure equal treatment for all patients, irrespective of their background.
Explainable AI techniques for Transparency and interpretability
Utilise explainable AI techniques that provide clear explanations and justifications for AI-generated decisions, enhancing trust and allowing healthcare professionals to validate and understand the reasoning behind AI recommendations.
For instance, in a cancer diagnosis system, utilising explainable AI techniques such as rule-based models or decision trees can provide clear explanations for AI-generated decisions.
When the AI system recommends a particular treatment plan or identifies a tumour type, it can provide a step-by-step breakdown of the features and criteria that led to the decision.
This transparency can allow healthcare professionals to validate the system’s reasoning, gain insights into the diagnostic process, and ultimately enhance trust in the AI system’s recommendations.
Validation and human oversight
Establish rigorous validation processes for AI algorithms, involving healthcare professionals in the development and deployment stages to provide oversight, double-check results, and minimise errors.
For instance, In the development of an AI-powered radiology system, rigorous validation processes can be established by involving radiologists and healthcare professionals.
They can review a large sample of medical images, comparing the system’s diagnoses to their own assessments. This process can help identify any discrepancies or errors, enabling refinements and improvements to the AI algorithm.
By having healthcare professionals involved in the validation process, the system’s accuracy and reliability can be enhanced, minimising potential errors and ensuring that the AI technology aligns with the expertise and standards of the medical community.
Ethical frameworks and guidelines
Develop ethical guidelines and regulations that address the ethical dilemmas posed by AI in healthcare, including issues such as informed consent, accountability, and the preservation of human values and decision-making in critical healthcare decisions.
For example, in the deployment of AI chatbots for mental health support, ethical guidelines and regulations can mandate the requirement of explicit informed consent from users.
The guidelines can specify that the chatbot must clearly communicate its limitations, ensure human oversight in critical situations, and prioritise user safety and privacy to uphold accountability and preserve human-centric values in mental healthcare.
Continuous monitoring and evaluation
Implementing robust monitoring systems to track and assess the performance and impact of AI applications in healthcare is critical, allowing for timely identification and resolution of any emerging risks or issues.
For instance, in an AI-driven clinical decision support system, a robust monitoring system can be implemented to continuously track the system’s performance and impact.
It can analyse real-time patient outcomes, compare AI recommendations to healthcare professionals’ decisions, and identify any deviations or adverse effects. This enables prompt identification and resolution of emerging risks or issues, ensuring patient safety and the optimization of AI technology in healthcare.
Merit Data & Technology: A Trusted Web Scraping & Data Mining Partner, With a Deeply Ethical Approach
At Merit Data & Technology, our team of data scientists have extensive, in-depth experience in working with data to facilitate web scraping in an efficient and effective manner.
Our data scientists understand your data needs and create customised tools to deliver the right data in the format you need. They scale up and scale down the data collection process based on your business needs and validate data quality before it is used for analytics and decision-making with the help of AI ML tools partnered with careful human calibration.
To know more about our web scraping technologies and practices, visit https://www.meritdata-tech.com/data/
Related Case Studies
-
01 /
Document Collection and Metadata Management System For the Pharmaceutical Industry
A leading provider of data, insight and intelligence across the UK healthcare community needed quick and reliable access to a vast number of healthcare documents that are published everyday in the UK healthcare community.
-
02 /
Formularies Data Aggregation Using Machine Learning
A leading provider of data, insight and intelligence across the UK healthcare community owns a range of brands that caters to the pharmaceutical sector and healthcare professionals in the UK.