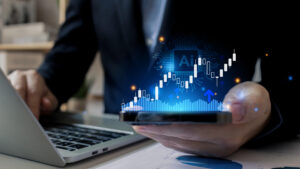
Artificial Intelligence (AI) is no longer a futuristic ambition; it has become a core driver of business transformation. From automating workflows and optimising customer experiences to uncovering deep insights from data, enterprises across industries are investing heavily in AI to gain a competitive edge. However, scaling AI from a handful of use cases to a pervasive, enterprise-wide capability presents significant challenges—ranging from integration complexities and talent shortages to governance and cost constraints.
Many organisations struggle to move beyond initial pilots/prototypes or isolated AI initiatives. The transition from proof-of-concept to large-scale implementation requires a well-structured strategy, addressing both technical and business considerations. Without the right foundation, AI deployments risk inefficiencies, fragmented adoption, and even failure to deliver meaningful ROI.
Despite the challenges surrounding AI adoption, 42% of enterprise-scale companies have actively deployed AI in their operations. These organisations have overcome obstacles by investing in training programs, adopting robust data governance frameworks, and integrating AI with existing systems to enhance efficiency and innovation. If you’re one of them, this is a great start. In this article, we provide key best practices to help your enterprise effectively scale up AI, unlocking even greater potential and benefits.
In this blog, we explore ten key best practices that can help enterprises successfully scale AI adoption. Whether you are looking to operationalise AI across business functions, optimise AI-driven decision-making, or improve governance and compliance, these insights will guide your journey. From aligning AI initiatives with business objectives to establishing robust LLMOps and fostering a data-driven culture, this guide provides a roadmap for enterprises to maximise their AI investments effectively.
Taking AI to the Next Level
Scaling AI for enterprises means moving beyond initial experiments to widespread, impactful use and leveraging AI to improve ROI. It involves integrating AI into core business processes, ensuring seamless data flow, and fostering a culture that embraces AI.
For enterprises, scaling AI is not just about expanding its use cases—it’s about ensuring seamless transition from prototype to production while maintaining performance, reliability, and business value. Many AI initiatives begin as isolated proofs of concept (PoCs) but fail to scale due to challenges like model drift, lack of infrastructure readiness, or misalignment with business workflows or incompatibility with customer environments. To succeed, organisations must focus on operationalising AI with robust MLOps pipelines, optimising deployment strategies, and ensuring continuous model monitoring and improvement.
Moreover, expanding AI across departments requires overcoming data silos, ensuring interoperability between AI systems, and fostering collaboration between technical and business teams. AI adoption should not remain confined to a single function—by integrating AI-driven decision-making into finance, supply chain, marketing, and other critical areas, enterprises can unlock enterprise-wide efficiencies and drive competitive advantage.
Additionally, addressing scalability and performance ensures that AI systems can handle increasing data volumes efficiently. Ensuring data security and compliance with regulations is essential to protect sensitive information and maintain trust. Lastly, measuring ROI through clear metrics demonstrates the value and impact of AI initiatives, justifying further investment and support.
10 Best Practices for Scaling Up AI in Enterprises
- Start Small, Scale Gradually
Begin with pilot projects to test AI’s viability and impact in specific areas. Use these early successes to build momentum and gradually expand AI applications across the organisation. This approach allows you to identify potential challenges and address them before full-scale deployment.
- Focus on Data Quality
Ensure your data is clean, accurate, and relevant. Implement robust data governance practices to maintain high data quality and integrity, which are crucial for effective AI performance. High-quality data is the foundation upon which AI systems make accurate predictions and decisions.
- Integrate with Existing Systems
Seamless integration with existing IT infrastructure is key. AI tools should easily access and utilise current data sources while complementing existing workflows. Successful integration allows AI to provide real-time insights and optimise decision-making.
- Ensure Scalability
Optimise AI models for scalability as data volume and processing demands grow. Choose scalable architectures, cloud-based solutions, and MLOps frameworks to ensure seamless model deployment, monitoring, and performance optimisation at scale.
- Balance Performance and Cost
Scaling AI efficiently requires optimising both cost and performance. Techniques such as model quantization, LoRA, QLoRA, and distillation help reduce computational overhead while maintaining accuracy. Consider cloud vs. on-premises deployment based on business needs.
- Prioritise Security and Compliance
AI applications often handle sensitive data, making security and regulatory compliance a top priority. Implement strong encryption, regular vulnerability assessments, and compliance checks to avoid reputational and legal risks.
- Measure ROI and Impact
Define clear KPIs to track AI’s financial and operational impact. Demonstrating ROI helps justify ongoing AI investments and refine AI strategies.
- Scale AI Responsibly
As AI expands across departments, bias, fairness, and ethical considerations must be embedded into AI governance. Ensuring explainability, auditability, and human oversight is crucial to prevent unintended consequences. Regulations like the EU AI Act require organisations to monitor AI risks, particularly in high-stakes industries like finance and healthcare.
- Encourage Continuous Improvement
AI is a rapidly evolving field. Enterprises must stay updated with the latest advancements and continuously refine AI models to adapt to new data patterns, business needs, and compliance requirements. A mindset of continuous learning and model retraining ensures that AI remains competitive and relevant.
- Data Foundation is Key to AI Success
The success of AI depends on high-quality, structured, and well-governed data. AI models trained on inconsistent or biased data will generate unreliable results. Establishing strong data collection, storage, and management practices is fundamental to improving model accuracy and business outcomes. Without a solid data foundation, even the most sophisticated AI solutions will fail to deliver value.
Harnessing AI for Future Growth
Keep in mind that scaling up AI in your enterprise involves strategic planning and careful execution. Focus on pilot projects, ensuring data quality, and fostering an AI-centric culture. Integrate AI with existing systems seamlessly, and prioritise scalability, security, and compliance. Regularly measure ROI and encourage continuous improvement to stay ahead. Avoid rushing the process and ensure robust governance and ethical considerations.
The potential of AI in the future for enterprises is immense. AI will drive continuous innovation, enhance decision-making, and improve efficiencies by automating tasks and providing personalised customer experiences. It offers predictive analytics, scalability, and new revenue streams while promoting sustainability. By adhering to best practices, organisations can unlock the full potential of AI, leading to sustainable growth and competitive advantage.
Merit – A Trusted AI & ML Development Partner
Merit engineers the data that powers the next generation of AI and technology. By providing bespoke data solutions, we combine proven technologies with human expertise to fuel the success of intelligence-driven businesses. Our innovation hub, MeritLABS, incubates cutting-edge technologies in AI, robotics, ML, and big data processing, helping clients harness disruptive solutions for real-world impact.
We deliver end-to-end AI/ML solutions designed to automate business processes, optimise ROI, and enhance efficiency. Our Natural Language Processing (NLP) systems extract valuable insights from unstructured data, enabling businesses to unlock hidden opportunities in blogs, documents, and more. Supported by advancements in deep neural networks, semantic architecture, knowledge graphs, and data mining, our AI and data analytics solutions empower businesses to maximise value and make data-driven decisions. Whether in large-scale automation or refined data insights, Merit ensures you stay ahead in the evolving tech landscape.
Key Takeaways
- Start small, validate AI’s impact, and scale strategically across the enterprise.
- High-quality data is non-negotiable—invest in governance and integrity.
- Ensure AI integrates smoothly with existing workflows and IT systems.
- Scalability is crucial; adopt MLOps and infrastructure that support growth.
- Optimise AI’s cost-performance ratio with efficient modeling techniques.
- Security and compliance must be built into AI initiatives from day one.
- Establish clear metrics to measure AI’s ROI and long-term business value.
- AI expansion should be ethical, responsible, and aligned with regulations.
- AI models must continuously evolve to remain accurate and relevant.
- A strong data foundation determines AI’s success—prioritise it.
Related Case Studies
-
01 /
Advanced ETL Solutions for Accurate Analytics and Business Insights
This solutions enhanced source-target mapping with ETL while reducing cost by 20% in a single data warehouse environment
-
02 /
Enhancing News Relevance Classification Using NLP
A leading global B2B sports intelligence company that delivers a competitive advantage to businesses in the sporting industry providing commercial strategies and business-critical data had a specific challenge.