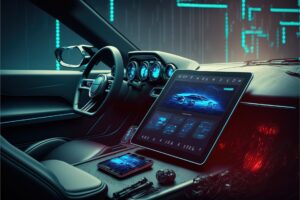
AI adoption in the global automotive industry has witnessed significant growth, revolutionising various aspects of vehicle development, manufacturing, and driving. If we had to summarise it, three significant trends have shaped this transformation. First among them is the transition from internal combustion vehicles to electric vehicle technology. EV manufacturers across Asia, China, Europe and the United States have successfully implemented R&D process innovations, gaining strategic advantage over established players. The second big transformation is, automotives increasingly focusing on software applications such as infotainment and advanced driver-assistance systems. Lastly, despite being in its early stages, a keen interest in Generative AI, which promises a radically different research & development future. Recent reports show that 75% of automotive companies are experimenting with at least one Gen AI application, while the remaining 25% plan to adopt it within a year.
These trends tell us that global automotives are committed to leveraging AI’s potential to make businesses more efficient, create value for end customers, and develop a competitive advantage. With that said, we take a deep dive into current AI use cases in the global automotive industry, and how automotive are capitalising on them.
AI in Autonomous Vehicles
Autonomous vehicles, or self-driving cars rely on a combination of sensors, artificial intelligence, and advanced software to navigate roads without human input. These cars use various sensors such as radar, LiDAR, video cameras, and ultrasonic sensors to gather information about the environment, including nearby objects, road signs, pedestrians, and other vehicles. For instance, GPS systems provide the precise geographic location of the vehicle (longitude, latitude, and elevation). They help the car understand its position on the map. And, the ultrasonic sensors detect objects at close range. They are used for emergency brake assistance, and for checking blind spots. The car’s AI systems process the collected data, the machine learning algorithms analyse real-world scenarios, and the car makes decisions based on this processed information. Every autonomous vehicle has a backup system. If the primary AI fails, the backup system (which ranges from manual driving, to automatic emergency braking) takes over.
According to data, in 2019, there were 31 million cars on roads with some level of automation, a number that is set to touch 54 million in 2024. Companies like Tesla have raised USD 20.2 billion in funding to build autonomous vehicles, whereas Audi has spent close to USD 16 billion self-driving cars last year. As competition intensifies, investments in autonomous vehicles are only set to rise rapidly. In fact, companies are now investing in infrastructure to facilitate AV production and adoption.
Advanced Driver Assistance Systems (ADAS)
ADAS, known as Advanced Driver Assistance Systems, operates through a sophisticated network of sensors, cameras, and radars to enhance driver safety and mitigate collision risks. Sensors continuously gather real-time data, detecting objects, measuring distances, and identifying potential hazards. This network includes ultrasonic sensors for parking assistance, LiDAR sensors for advanced functionalities like adaptive cruise control, and radar sensors, particularly valuable in adverse weather conditions. High-resolution cameras play a pivotal role in providing visual insights, detecting lane markings, traffic signs, pedestrians, and other vehicles. The control unit processes this amalgamated data using intricate algorithms to detect potential hazards and make informed decisions, such as issuing warnings or initiating emergency braking. ADAS offers a range of safety features, including lane departure warnings, automatic emergency braking, and adaptive cruise control, aiming to diminish human errors that lead to accidents.
With substantial investments from leading original equipment manufacturers (OEMs) and incentives like insurance premium discounts for ADAS-equipped vehicles, the global ADAS market is witnessing rapid growth. According to reports, the global ADAS market size was estimated at USD 30.9 billion in 2022 and is projected to reach USD 65.1 billion by 2030 (CAGR of 9.7% during 2022-2030). Furthermore, ADAS technologies pave the way for the transition to autonomous vehicles by simplifying driving complexities and bolstering safety measures.
Predictive Maintenance
Predictive maintenance is a game-changer in the automotive industry, and artificial intelligence (AI) plays a pivotal role in making it effective. Equipped with numerous sensors and onboard computers, modern vehicles continuously gather data on various parameters such as engine performance, brake wear, battery health, and tire pressure. AI algorithms analyse this data to establish normal behavior patterns for different components, flagging deviations as anomalies that may indicate potential failures. Utilising machine learning techniques like regression and deep learning, predictive algorithms forecast when specific parts might fail based on factors such as usage patterns, environmental conditions, and wear-and-tear.
For instance, AI predicts engine component malfunctions by analysing factors like RPM, temperature, and oil quality, while brake pad life is estimated through braking patterns and wear rates. Battery health is tracked using data on voltage, charge cycles, and temperature. This proactive approach to maintenance allows for timely intervention, minimising unexpected breakdowns and reducing vehicle downtime.
Predictive maintenance not only saves costs by addressing issues before they escalate but also prevents catastrophic failures that could lead to expensive repairs or accidents. Efficient maintenance schedules optimised by AI further contribute to long-term cost savings. In commercial fleet management, AI-driven predictive maintenance is particularly crucial. Fleet managers can remotely monitor multiple vehicles, with predictive models aiding in resource allocation to ensure timely maintenance for all vehicles.
A Merit expert says, “AI adoption in the automotive industry is revolutionising vehicle development, manufacturing, and driving experiences. From electric vehicles to autonomous driving, AI is reshaping the future of mobility.”
Natural Language Processing in Infotainment Systems
NLP technology enables voice assistants to understand and interpret human language with high accuracy. By analysing speech patterns, syntax, and context, NLP algorithms decipher user commands and queries effectively. This leads to a more seamless and natural interaction between users and voice assistants. NLP allows hands-free control of various functions like adjusting climate settings, changing radio stations or playlists, and setting navigation destinations. NLP also tailors responses based on user preferences and context.
The NLP market was valued at USD 11.21bn in 2022, and it is projected to reach USD 49.79bn by 2029. Two foremost trends we’re seeing in NLP are, automotives are investing in NLP-powered voice assistants to enhance user experience and drive business growth. Whereas OEMs are collaborating with tech giants to integrate advanced NLP features in infotainment systems.
Intelligent Traffic Management System & Enhanced Navigation Systems
Intelligent Traffic Management (ITM) revolutionises urban mobility by harnessing real-time data from cameras, sensors, and GPS to optimise traffic flow and ensure safety. AI algorithms analyse this data to predict congestions and dynamically adjust traffic signals, lane assignments, and speed limits, thus preventing accidents and hazardous conditions. In Konya, Turkey, for example, ITM implementation resulted in significant reductions in carbon emissions and wait times, showcasing its effectiveness in tackling modern traffic challenges and enhancing road efficiency.
Enhanced Navigation Systems
Enhanced navigation systems powered by AI provide personalised, real-time route optimisation and guidance to users. These systems consider various factors such as traffic conditions, weather, and user preferences to dynamically adjust routes and suggest faster or more scenic options. Predictive features anticipate arrival times, traffic bottlenecks, and even charging station locations for electric vehicles, ensuring seamless and efficient navigation experiences. With global adoption on the rise, companies invest in map data, machine learning, and cloud infrastructure to integrate AI-enhanced navigation seamlessly with voice assistants, further enhancing user convenience and satisfaction.
Digital Twins
Digital twins in the automotive industry are virtual replicas of cars, holding real-time data on performance, inspections, and service history. They’re used in various ways, like testing products to enhance their quality and performance. Engineers can experiment virtually with different materials and designs, such as testing new tires for different weather conditions. Companies also use digital twins to plan manufacturing capacity by simulating the impact of new machines on production. They’re even used for employee training, allowing workers to learn remotely before equipment is physically installed. Additionally, digital twins predict maintenance needs for machines and equipment, improving the health of production lines. Finally, they provide insights for sales by allowing customers to explore products before release, informing sales strategies and product improvements.
Personalised Customer Experience
Automotive companies gather a lot of data from different places like customer profiles, vehicle sensors, online activities, and service records. They use AI algorithms, like collaborative filtering and regression models, to process this data. These algorithms make personalised recommendations, like suggesting vehicle configurations or predicting maintenance needs. For example, based on a customer’s preferences and vehicle data, AI can recommend the right color or when it’s time for an oil change. It even helps with financing plans by considering a customer’s financial history and budget. Plus, AI learns from user interactions in real-time, adapting recommendations accordingly, like suggesting electric vehicles if a customer shows interest. This ensures that suggestions are always up-to-date and tailored to each customer’s needs.
The Future of AI in Automotive
The global automotive industry is witnessing a profound transformation fueled by AI adoption. Electric and autonomous vehicles are at the forefront, with AI optimising performance and safety while facilitating the transition from internal combustion engines to electric power. Moreover, vehicles are evolving into software-defined entities, empowering advanced features and over-the-air updates. Gen AI is revolutionising R&D processes, enabling faster innovation and strategic advantages in time-to-market. Around 75% of automotive companies are already experimenting with AI applications, reflecting the industry’s recognition of its potential value. AI-driven product testing will simulate real-world scenarios, enhancing customisation options for consumers. Predictive maintenance, facilitated by AI, will prevent breakdowns and improve safety, particularly in autonomous vehicles. Ultimately, AI will reshape the entire automotive business model, driving efficiency, cost reduction, and innovation across manufacturing and sales processes.
Merit’s Expertise in Data Aggregation & Harvesting for the Global Automotive Sector
Merit Data and Technology excels in aggregating and harvesting automotive data using AI, ML, and human expertise. Our capabilities include:
- Crafting end-to-end data pipelines and scalable data warehouses
- Designing compliant governance solutions for seamless integration
- Utilising high-volume, high-velocity data tools for nuanced insights
- Extracting retail product attributes and audience data
- Aggregating industry-specific data points for informed decision-making
Trusted by leading automotive brands, Merit drives innovation and efficiency by delivering refined, actionable insights.
Key Takeaways
- AI Adoption in Automotive: AI adoption in the automotive industry has seen significant growth, revolutionising vehicle development, manufacturing, and driving experiences.
- Key Trends Shaping Transformation: Three significant trends have shaped this transformation: the transition to electric vehicles, the increasing focus on software applications, and the rising interest in Generative AI.
- Autonomous Vehicles: AI plays a crucial role in autonomous vehicles, enabling them to navigate roads without human input through sensors, AI systems, and backup mechanisms.
- Predictive Maintenance: AI-driven predictive maintenance analyses vehicle data to forecast component failures, preventing breakdowns and reducing downtime.
- Intelligent Traffic Management & Navigation: AI optimises traffic flow and enhances safety through intelligent traffic management systems. Enhanced navigation systems provide personalised, real-time route optimisation and guidance to users.
- Digital Twins & Personalised Customer Experience: Digital twins replicate vehicles in virtual space, aiding in product testing, manufacturing planning, and sales insights. AI algorithms also provide a personalized customer experience, making recommendations and predicting maintenance needs based on customer data.
Related Case Studies
-
01 /
A Hybrid Solution for Automotive Data Processing at Scale
Automotive products needed millions of price points and specification details to be tracked for a large range of vehicles.
-
02 /
A Digital Engineering Solution for High Volume Automotive Data Extraction
Automotive products required help to track millions of price points and specification details for a large range of vehicles.