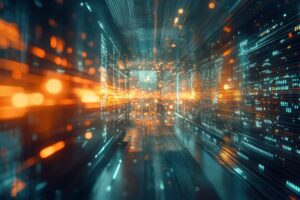
Introduction: The Need for AI-Ready Data Platforms
In an era where businesses depend on analytics, AI and machine learning for decision-making, legacy data platforms often fail to keep pace with modern demands. Outdated systems struggle with scalability, integration, and flexibility, leaving businesses unable to fully capitalise on emerging technologies. Transitioning to modern data solutions will lay the foundation to build next-generation systems with AI, analytics and data at the core of business processes. According to this Forrester Wave Report, 74% of global CIOs report that they already have a lakehouse in their technology estate, underscoring the growing reliance on this architecture for modern business needs.
This blog explores how adopting a lakehouse architecture can transform data platforms into AI-ready ecosystems, supported by Merit’s proven modernisation methodologies and industry-aligned frameworks.
Architecture Patterns for Modern Data Management
From a data management perspective, modern systems are leveraging the following technologies to enhance AI and machine learning capabilities:
- Data Lakes: Traditionally, data lakes have served as cost-effective storage for raw, unstructured data. However, their lack of governance and structure makes AI model training and real-time inferencing challenging. Organisations looking to build AI-ready platforms must implement metadata-driven governance layers on top of data lakes to enable efficient data retrieval and AI/ML pipeline automation.
- Lakehouses: A lakehouse architecture enhances AI-powered platforms by combining the scalability of data lakes with the reliability and performance of warehouses. Lakehouses support ACID transactions, which are critical for AI feature stores that enable real-time model training and inference. Additionally, they allow structured and unstructured data to coexist seamlessly, making it easier to perform complex, database-like queries for AI workloads.
- Data Mesh: Data mesh decentralises data ownership and enables domain-oriented teams to manage data as a product. From an AI perspective, this empowers data scientists and ML engineers to work independently within their domains, allowing them to create sandboxed environments for experimentation and training AI models without disrupting enterprise-wide data pipelines. This results in greater agility and faster time-to-value for AI initiatives.
- Data Fabric: A data fabric serves as an intelligent data management and integration framework, ensuring real-time access to data from multiple sources. AI systems thrive on fresh, high-quality data, and data fabric architectures reduce data duplication while enabling consistent, on-demand access to AI-ready datasets. By integrating governance, security, and automation, data fabrics enhance AI pipelines, ensuring data integrity and faster model deployments.
By structuring modern architectures with AI fitment in mind, organisations can accelerate their AI/ML initiatives, streamline data access, and create robust foundations for predictive and prescriptive analytics.
Lakehouse Strategies That Deliver Tangible ROI
Adopting a lakehouse strategy offers several benefits that directly impact business performance and AI enablement:
- Scalability and Cost Efficiency
- Cloud-native lakehouses dynamically scale to handle growing data volumes without exorbitant costs, which is critical for AI systems.
- Unified storage eliminates the need for expensive ETL processes, reducing operational expenses. This is especially important as AI models are using more and more data.
- Improved Data Accessibility and Collaboration
- Lakehouses centralise data access, enabling both structured and unstructured data to be used across teams and workflows.
- AI-specific governance capabilities, such as row-level (RL) or column-level (CL) access controls, ensure that teams can securely access and manipulate datasets.
- Supports the creation of structured and secure sample datasets for AI/ML training across different teams and departments, ensuring compliance with data governance policies, facilitating seamless collaboration between data scientists and engineers.
- Faster AI/ML Workflows
- Lakehouses integrate seamlessly with a wide range of AI/ML tools, including ETL pipelines, GUI-based tools, and programmatic libraries such as TensorFlow, PyTorch, and Scikit-learn.
- Real-time data access and streaming capabilities allow AI models to be continuously retrained, improving model accuracy and operational efficiency.
- Reduced Time-to-Market
- Cloud-based lakehouse platforms, such as Azure Fabric and Databricks, provide pre-trained AI models and industry-specific templates that accelerate development.
- Standardised AI-ready frameworks reduce the time required for model deployment, enabling businesses to derive insights and automation benefits more rapidly.
Lakehouses are continuously evolving to stay relevant to modern business needs. For instance, leading providers like Databricks are updating their product strategies to empower the unification of lakehouse architectures with Generative AI capabilities. This evolution ensures that businesses can leverage the synergy between large-scale data processing and generative AI workflows to gain actionable insights faster and more effectively.
Data Engineering: The Backbone of AI-Ready Platforms
Data engineering plays a pivotal role in the success of AI-ready platforms, ensuring data is organised, accessible, and primed for analysis. Robust data engineering practices form the foundation for modern architectures like lakehouses, enabling seamless integration with AI/ML workflows. Here are some key considerations:
- Data Integration and Ingestion: Efficiently ingest data from diverse sources, including structured databases, APIs, and unstructured files, into a unified platform.
- Data Transformation: Employ ETL/ELT pipelines to clean, format, and enhance raw data, making it analytics-ready.
- Real-Time Data Streaming: Enable real-time data processing to support AI-driven insights and immediate decision-making.
- Metadata Management: Ensure comprehensive metadata tracking to improve data governance and discoverability.
- Feature Engineering: Develop and manage feature stores that allow AI models to be trained on high-quality, curated features, improving predictive accuracy and model performance.
- Vector Databases: Enable AI applications like search and recommendation engines by utilising vector databases for similarity search, semantic retrieval, and embedding-based queries.
- Data Governance: Implement robust data access policies, lineage tracking, and compliance measures to ensure AI models are trained on high-quality, bias-free, and secure data.
By investing in advanced data engineering capabilities, organisations can unlock the full potential of their data, reducing silos and enabling actionable insights across all business functions. Data engineering not only supports scalability but also enhances the agility and efficiency of AI-driven processes.
Merit: A Preferred Partner for Data Lakehouse Implementations
Merit specialises in creating AI-ready platforms using its AI-first architecture frameworks and proven modernisation methodologies. Here’s how Merit helps organisations unlock the full potential of lakehouse strategies:
- AI-Ready Architecture Frameworks:
- Designed to migrate legacy platforms to modern solutions such as lakehouses and data meshes, ensuring alignment with organisational goals.
- Templates and tools accelerate implementation while maintaining best practices.
- Industry-Agnostic and Cloud-Native Solutions:
- Merit’s solutions are designed for flexibility and compatibility across industries and leading cloud ecosystems, making modernisation seamless.
- Integrated DevSecOps Framework:
- Automates deployment pipelines and CI/CD processes, enhancing operational efficiency and governance.
- Test Automation and Efficiency Gains:
- Automated test workflows ensure comprehensive coverage, reducing defects and effort while maintaining high-quality standards.
- Validation processes are faster and more reliable, leading to a 40–70% reduction in testing efforts.
Customer Journey: From Legacy to Lakehouse
A Merit customer, an automotive industry intelligence pioneer, was struggling with slow decision-making due to siloed legacy systems. The sector was getting disrupted with next-generation AI and advanced analytics capabilities, and the company had to act fast.
By transitioning to a lakehouse architecture with Merit’s frameworks:
- Infrastructure costs were reduced by 35% by robust cloud infrastructure optimisation.
- Data query speeds improved by 50%, enabling faster insights.
- Machine learning models were operationalised, driving a significant increase in customer satisfaction scores.
These results demonstrate how a lakehouse strategy is central to transforming legacy systems into AI-ready platforms that deliver measurable business outcomes.
Steps to Build an AI-Ready Lakehouse Platform
Building an AI-ready platform requires a strategic approach. Here’s how Merit’s methodologies guide the process:
- Assess Current Architecture:
- Conduct a comprehensive evaluation of legacy systems to identify bottlenecks and inefficiencies.
- Future-Ready System Design:
- Leverage Merit’s AI-Ready Architecture Framework to identify, and design scalable and compliant platforms.
- Adopt Modern Data Management Platforms:
- Transition to lakehouse and data mesh solutions (or data fabric if needed) to support advanced analytics and AI workflows involving both raw data and structured data from other systems.
- Integrate Governance and Security:
- Embed governance tools and security measures to ensure compliance and data integrity. Plan for data distribution and data access challenges.
- Monitor and Optimise:
- Use performance metrics and analytics to continually optimise the platform, aligning it with evolving business needs.
Conclusion: Transforming Data into a Strategic Asset
Lakehouse architectures enable businesses to intake data from disparate sources for model building and analytics, improve decision-making, and achieve measurable ROI.
Beyond analytics, companies are now looking for data intelligence platforms that go beyond traditional data needs and are ready to democratise data and AI across organisations at an unprecedented scale. By leveraging Merit’s proven methodologies and frameworks, businesses can build platforms that align with this new vision, unlocking the full potential of their data ecosystems while ensuring scalability and innovation.