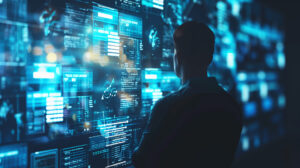
Since the early 2010s, the rise of the internet has led to an explosion of data, prompting companies like Google, Meta, and Amazon to build sophisticated data infrastructure to manage the immense data volume. Data engineering has since evolved significantly, driven by the widespread adoption of AI, cloud computing, edge computing, data privacy practices, and more.
According to a report by StartUs Insights, the data engineering industry has experienced a growth rate of 22.89% in the last year, with over 150,000 professionals employed in the sector. Moreover, organisations that have embraced advanced data engineering practices have reaped significant benefits, including improved operational efficiency, enhanced decision-making capabilities, and the ability to deliver personalised customer experiences.
In this article, we touch upon the key growth spurts data engineering has witnessed in the last few years, and trends that will define this space in 2025.
Evolution of Data Engineering in the Past Few Years
In the past few years, data engineering has evolved significantly, driven by advancements in technology and changing business needs. The explosion of big data has necessitated robust data infrastructure to store, process, and analyse vast amounts of information. Technologies such as cloud computing, machine learning, and real-time data processing have played a crucial role in this transformation.
Cloud Computing: The rise of cloud services like AWS, Google Cloud, and Microsoft Azure has reshaped the way organisations handle large datasets. This shift has made data management more scalable and cost-effective, enabling companies to handle vast amounts of information with greater flexibility.
Machine Learning and AI: Integrating AI and machine learning into data processes has automated many tasks, improved data quality and delivering deeper insights. As a result, the demand for skilled data engineers has surged, with companies seeing a 15% increase in revenue growth by turning raw data into actionable insights.
Real-Time Data Processing: The need for real-time analytics has spurred the development of technologies for streaming data. This capability allows businesses to make faster, data-driven decisions, enhancing operational efficiency by up to 20%. Companies can respond quickly to changes, staying ahead in competitive markets.
Data Privacy and Security: Due to regulations like GDPR and CCPA, data privacy and security have become top priorities. Data engineers are now essential in ensuring compliance and protecting sensitive information, which is crucial for maintaining customer trust.
Data Quality Improvement: Effective data engineering has led to a 25% improvement in data quality. High-quality data is vital for accurate analytics and reliable business intelligence, enabling organisations to make informed decisions.
Customer Satisfaction: By leveraging data engineering to personalise customer experiences, companies have seen a 30% increase in customer satisfaction. Analysing customer data helps businesses in tailoring their services to meet individual preferences.
Cost Savings: Organisations implementing data engineering practices have achieved cost savings of up to 18%. Streamlined data management reduces manual intervention and minimises errors, leading to more efficient operations.
Job Market Demand: The demand for data engineering skills has created a surge in job openings, with over 31,000 data engineer vacancies currently listed on job platforms such as Indeed. This trend highlights the critical role data engineering plays in fostering data-driven decision-making and innovation.
Trends Defining Data Engineering in 2025
The trends we can expect in 2025 are a natural evolution of the advancements we have already seen in data engineering in recent years. Technologies like cloud computing, AI, and real-time data processing have laid the foundation for more sophisticated practices.
For instance, the rise of cloud services made it easier to manage large datasets, setting the stage for cloud-native technologies that enhance scalability and flexibility. Similarly, AI and machine learning, which have automated many data processes, are now being integrated even more deeply, driving automation and predictive analytics further.
The push for real-time analytics, which began with the need for faster data processing, is now evolving into edge computing, allowing data to be processed closer to its source. This reduces latency and improves response times, particularly critical for IoT applications.
Moreover, the importance of data privacy and security that emerged with regulations like GDPR and CCPA continues to be a priority, leading to advanced practices and technologies to protect sensitive information.
With that said, let’s dive into what we can expect from the coming year.
DataOps and DevOps: Enhancing collaboration and speed by using agile methods to simplify data tasks and improve teamwork between data engineers, data scientists, and business teams. These methodologies promote efficient data management, continuous integration, and delivery of data solutions, ensuring agility and reliability in data projects.
Edge Computing: Driving real-time data analytics by processing data closer to where it’s created, reducing latency and improving response times. This trend will enhance data security and enable faster decision-making, benefiting industries like IoT and autonomous vehicles.
AI and ML Integration: Leveraging AI and machine learning to automate data processes, improve data quality, and provide deeper insights. Advanced AI models will offer predictive analytics and streamline data workflows, reducing manual intervention and allowing data engineers to focus on strategic tasks.
Cloud-Native Technologies: Adopting cloud-native solutions to enhance scalability, flexibility, and cost-efficiency in data management. This approach will facilitate seamless data integration and processing, making it easier to manage large datasets.
Reducing Data Silos: Breaking down data silos to ensure seamless data flow and accessibility across different departments and systems. Integrated data environments will enable more comprehensive analysis and better-informed decision-making.
Generative AI: Using generative AI models to create synthetic data, automate data labelling, and uncover patterns in large datasets. This technology will enhance data diversity, improve model training, and offer new insights into data.
Data Privacy and Security: Increasing focus on data privacy and security measures to comply with regulations and protect sensitive information. Robust security protocols and compliance strategies will be essential in maintaining customer trust and safeguarding data.
Real-Time Data Processing: Implementing real-time data processing capabilities to enable instant decision-making and improve operational efficiency. This trend will support faster response times and more dynamic business operations.
Serverless Data Engineering: The rise of serverless architectures will enable data engineers to build and deploy data pipelines without managing underlying infrastructure. This approach offers scalability, cost-effectiveness, and ease of maintenance, allowing organisations to focus on data processing rather than infrastructure management.
Data Lakes: Becoming more sophisticated, data lakes will provide a unified repository for structured and unstructured data. Advanced data lake technologies will offer better data governance, security, and integration with other data platforms, enabling organisations to extract valuable insights from diverse data sources.
To summarise, the evolution of data engineering over the past few years has been driven by rapid advancements in technology and growing business needs. As we look toward 2025, trends like DataOps, edge computing, and the integration of AI will further enhance data management practices. With an emphasis on real-time processing and robust data privacy measures, organisations are poised to unlock deeper insights and foster innovation. Embracing these trends will be crucial for businesses aiming to remain competitive in a data-driven landscape, ensuring they can leverage their data assets effectively and responsibly.
Merit’s Expertise in Data Aggregation & Harvesting Using AI/ML Tools
Merit’s proprietary AI/ML tools and data collection platforms meticulously gather information from thousands of diverse sources to generate valuable datasets. These datasets undergo meticulous augmentation and enrichment by our skilled data engineers to ensure accuracy, consistency, and structure. Our data solutions cater to a wide array of industries, including healthcare, retail, finance, and construction, allowing us to effectively meet the unique requirements of clients across various sectors.
Our suite of data services covers various areas: Marketing Data expands audience reach using compliant, ethical data; Retail Data provides fast access to large e-commerce datasets with unmatched scalability; Industry Data Intelligence offers tailored business insights for a competitive edge; News Media Monitoring delivers curated news for actionable insights; Compliance Data tracks global sources for regulatory updates; and Document Data streamlines web document collection and data extraction for efficient processing.
Key Takeaways
Rapid Growth in Data Engineering: The industry has grown by 22.89% over the past year, with over 150,000 professionals currently employed.
Benefits of Advanced Practices: Organisations utilising advanced data engineering enjoy improved operational efficiency, enhanced decision-making, and personalised customer experiences.
Technological Advancements: Key technologies driving this evolution include cloud computing, AI, machine learning, and real-time data processing, which enable better data management and insights.
Focus on Data Privacy: Compliance with regulations like GDPR and CCPA is essential, highlighting the importance of data privacy and security in data engineering.
Improved Data Quality: Effective data engineering has led to a 25% improvement in data quality, essential for reliable business intelligence.
Customer Satisfaction Boost: Personalising customer experiences through data analysis has resulted in a 30% increase in customer satisfaction.
Emerging Trends for 2025: Key trends include DataOps and DevOps for enhanced collaboration, edge computing for real-time analytics, and the continued integration of AI and machine learning.
Serverless Architectures: Serverless data engineering will simplify pipeline management, focusing more on data processing than infrastructure.
Unified Data Repositories: The evolution of data lakes will facilitate better governance and integration of diverse data sources.
Job Market Demand: The growing need for skilled data engineers is reflected in over 31,000 job vacancies, emphasising the critical role of data engineering in business innovation.
Related Case Studies
-
01 /
A Digital Engineering Solution for High Volume Automotive Data Extraction
Automotive products required help to track millions of price points and specification details for a large range of vehicles.
-
02 /
Automated Data Solution For Curating Accurate Regulatory Data At Scale
Learn how a leading regulatory intelligence provider is offering expert insights, analytics, e-Learning, events, advisory and consulting focusing on the payments and gambling industries