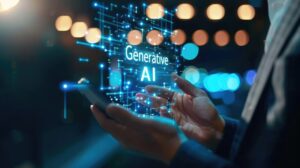
Generative AI (GenAI) has revolutionised the enterprise landscape, offering innovative solutions and capabilities that drive efficiency, creativity, and personalised customer experiences. Unlike traditional AI, which relies on predefined rules and datasets, GenAI can generate new content, ideas, and solutions autonomously. This transformative potential is reshaping industries, from healthcare to finance to entertainment.
In this article, we delve into five unique requirements that enterprises must address to successfully implement GenAI solutions. These requirements go beyond well-known platforms like ChatGPT, focusing on the critical infrastructure, models, security, integration, and scalability needed to harness the full potential of GenAI in the corporate world. Let’s explore these requirements in detail.
Requirement 1: Robust Data Infrastructure
A solid data infrastructure is the backbone of any successful GenAI implementation. For example, consider an e-commerce giant like Amazon. They handle vast amounts of data, both structured (such as product listings and customer profiles) and unstructured (such as customer reviews and browsing behaviour). To support GenAI effectively, Amazon relies on scalable storage solutions capable of managing this immense data volume.
This data must also be easily accessible, allowing AI systems to retrieve and process information efficiently. Netflix, for instance, uses an advanced data infrastructure to analyse viewing habits and preferences in real time, enabling them to recommend personalised content to users almost instantaneously.
Additionally, robust data integration capabilities are essential. Enterprises often gather data from various sources, including customer interactions, transactional records, and external databases. Integrating this data seamlessly is crucial. For example, a global retailer like Walmart integrates data from its online sales, physical stores, and third-party vendors to ensure their AI models have a comprehensive view of customer behaviour. This holistic data integration enables Walmart to generate accurate and relevant outputs, such as personalised shopping recommendations and optimised inventory management.
Requirement 2: Advanced Machine Learning Models
Cutting-edge machine learning models are essential for tailoring GenAI to meet the specific needs of enterprises. These models must be capable of learning from vast datasets and improving over time to deliver increasingly accurate and relevant outputs. For instance, consider Google Photos, which uses advanced image recognition models and tag users’ photos to organise automatically. This technology can handle complex tasks such as recognising faces, objects, and even specific scenes, making it indispensable for user-friendly experiences.
Domain-specific models are particularly valuable as they can be fine-tuned to address unique business requirements. For example, in the healthcare sector, IBM Watson is used to understand medical terminology and analyse patient data, providing insights that support clinical decision-making. These models are trained to handle the complexities of medical records, treatments, and outcomes, making them highly specialised and effective in improving patient care.
In the retail industry, companies like Amazon use models focused on customer behaviour and sales patterns to personalise shopping experiences. Their recommendation system analyses purchase history, browsing behaviour, and even demographic data to suggest products that a customer is likely to buy. This level of personalisation drives higher conversion rates and customer satisfaction.
The ability to customise and fine-tune these models ensures that enterprises can leverage AI to address their specific challenges and opportunities effectively. By deploying advanced machine learning models tailored to their industry, businesses can unlock new efficiencies, enhance customer experiences, and stay competitive in a rapidly evolving market.
Requirement 3: Enhanced Security and Compliance
Security and compliance are non-negotiable in the world of GenAI. Enterprises handle vast amounts of sensitive data, from customer information to proprietary business insights. To protect this data, stringent security measures must be in place. For instance, financial institutions like HSBC implement multi-layered security protocols, including encryption, access controls, and continuous monitoring, to safeguard their data.
Compliance with industry regulations and standards is equally critical. Regulations like the GDPR in Europe or HIPAA in the United States mandate specific protections for personal and health-related data. Companies like Pfizer, which deal with sensitive health data, ensure compliance by adhering to these regulations, implementing robust data governance practices, and conducting regular audits to maintain data integrity and privacy. Failure to comply can result in hefty fines and damage to reputation, making it imperative for enterprises to prioritise both security and compliance.
Requirement 4: Seamless Integration with Existing Systems
For GenAI to be effective, it must seamlessly integrate with existing enterprise software and workflows. This ensures that AI-driven insights and functionalities enhance rather than disrupt current operations. For example, Salesforce’s Einstein AI integrates smoothly with their CRM platform, allowing sales teams to gain AI-powered insights without changing their workflows.
Interoperability and API compatibility are key to this integration. A case in point is Microsoft’s Azure, which provides a wide range of APIs and supports multiple programming languages, enabling businesses to integrate GenAI solutions with their existing IT infrastructure. This ensures that data flows seamlessly between systems, enhancing productivity and enabling a unified approach to data management.
Requirement 5: Scalable Deployment and Management
Scalable deployment options are essential for enterprises utilising GenAI to accommodate growing data and user demands. As businesses expand, the volume of data processed increases exponentially. For example, streaming services like Spotify need to manage enormous amounts of user data, from listening habits to song preferences, which continuously grow as their user base expands. Scalable deployment ensures that the AI infrastructure can handle this growth without performance degradation.
Cloud-based solutions, such as those offered by AWS or Google Cloud, provide the necessary scalability. These platforms allow enterprises to dynamically allocate resources based on current demands, ensuring that the AI systems remain responsive and efficient. This scalability is crucial for maintaining a seamless user experience, even during peak usage times.
Efficient management tools and monitoring systems are equally important to maintain optimal performance. Tools like Kubernetes and Docker allow for the orchestration and management of containerized applications, making it easier to deploy, scale, and manage AI applications. For instance, companies like Airbnb use these tools to manage their large-scale AI-driven services, ensuring that their infrastructure remains robust and efficient.
Monitoring systems play a vital role in identifying and addressing performance issues before they impact the end-user experience. Solutions like Prometheus and Grafana provide real-time monitoring and alerting capabilities, helping enterprises keep their GenAI systems running smoothly. By continuously tracking performance metrics and system health, businesses can proactively manage their AI deployments and ensure they meet the required performance standards.
In conclusion, each of these elements is critical to unlocking the full potential of GenAI, ensuring efficient, secure, and effective AI-driven solutions. By addressing these requirements, enterprises can harness the power of GenAI to drive innovation, optimise operations, and deliver personalised experiences. Understanding and implementing these foundational aspects will position businesses to stay competitive and capitalise on the transformative capabilities of Generative AI.
A Merit expert quotes, “Scalability in AI solutions is not just an option; it’s a strategic necessity for growth.”
Merit’s Expertise in Data Aggregation & Harvesting Using AI/ML Tools
Merit’s proprietary AI/ML tools and data collection platforms meticulously gather information from thousands of diverse sources to generate valuable datasets. These datasets undergo meticulous augmentation and enrichment by our skilled data engineers to ensure accuracy, consistency, and structure. Our data solutions cater to a wide array of industries, including healthcare, retail, finance, and construction, allowing us to effectively meet the unique requirements of clients across various sectors.
Our suite of data services covers various areas: Marketing Data expands audience reach using compliant, ethical data; Retail Data provides fast access to large e-commerce datasets with unmatched scalability; Industry Data Intelligence offers tailored business insights for a competitive edge; News Media Monitoring delivers curated news for actionable insights; Compliance Data tracks global sources for regulatory updates; and Document Data streamlines web document collection and data extraction for efficient processing.
Key Takeaways
Robust Data Infrastructure: A strong data infrastructure is essential for handling vast amounts of structured and unstructured data efficiently.
Advanced Machine Learning Models: Cutting-edge, domain-specific machine learning models are crucial for delivering accurate and relevant outputs tailored to business needs.
Enhanced Security and Compliance: Implementing stringent security measures and adhering to industry regulations is vital to protect sensitive data and maintain trust.
Seamless Integration: GenAI solutions must integrate smoothly with existing systems and workflows to enhance productivity and avoid disruption.
Scalable Deployment and Management: Scalable infrastructure is necessary to accommodate growing data demands and ensure optimal performance, with tools for efficient management and monitoring.
Related Case Studies
-
01 /
High-Speed Machine Learning Image Processing and Attribute Extraction for Fashion Retail Trends
A world-leading authority on forecasting consumer and design trends had the challenge of collecting, aggregating and reporting on millions of fashion products spanning multiple categories and sub-categories within 24 hours of them being published online.
-
02 /
A Digital Engineering Solution for High Volume Automotive Data Extraction
Automotive products required help to track millions of price points and specification details for a large range of vehicles.