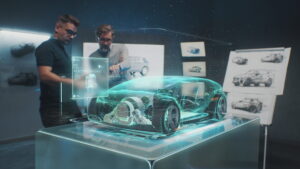
Automotive data encompasses a wide range of information crucial to the industry’s functioning. It includes vehicle specifications, maintenance records, history reports, fuel consumption data, telematics insights, market analysis, predictive maintenance, insurance underwriting, and fleet management. These diverse datasets empower automotive stakeholders to optimise operations, enhance vehicle performance, and anticipate market trends effectively.
7 Major Uses of Automotive Data
In recent times, automotive data has gained significant importance because it enables various transformative initiatives within the industry. Predictive maintenance has emerged as a key application, as automotive companies increasingly utilise data to forecast when vehicles require maintenance. By scrutinising patterns and performance metrics, they can foresee potential issues before they escalate into breakdowns, benefiting drivers with preemptive repairs and extending the lifespan of vehicle components. Moreover, improved vehicle performance is facilitated through data analytics, allowing manufacturers to optimise design and functionality. Analysis of data from sensors, cameras, and connected systems aids in enhancing safety, fuel efficiency, and overall driving experience.
Market insights derived from automotive data offer valuable perspectives into consumer behavior, preferences, and market trends. This empowers companies to tailor their offerings accordingly, resulting in better-targeted products and services. Additionally, the efficiency of supply chains is significantly bolstered through data-driven optimisation of inventory management, production schedules, and logistics, thereby reducing costs and enhancing productivity. With the automotive industry shifting towards Electric Vehicles (EVs), data plays a pivotal role in informing decisions regarding EV technology adoption, pricing strategies, and market selection. Understanding consumer preferences and charging infrastructure is essential for the successful integration of EVs.
The rise of connected cars further underscores the importance of automotive data. Telematics systems provide real-time insights into vehicle performance, driver behavior, and navigation, enabling personalised services and bolstering safety measures. Lastly, leveraging automotive data effectively grants companies a competitive advantage. Whether in product development, sales strategies, or aftermarket services, data-driven decisions consistently yield superior outcomes.
Challenges & Gaps Associated with Automotive Data Adoption
As cars evolve into highly sophisticated and connected entities, the deluge of data they generate presents a significant challenge. The influx of unstructured data from sensors, cameras, and various systems inundates the automotive ecosystem, complicating the extraction of meaningful insights. Moreover, the existence of Data Silos among different players in the automotive supply chain—such as Original Equipment Manufacturers (OEMs) and Tier 1 suppliers—restricts the effective utilisation of data across the industry, hampering collaboration and innovation.
Ensuring the quality and accuracy of data remains paramount, as flawed or incomplete data can lead to erroneous decisions, potentially impacting safety, performance, and customer satisfaction. Additionally, the issue of privacy and security looms large, necessitating a delicate balance between data utilisation and safeguarding sensitive information. Legacy systems further exacerbate challenges, with many automotive companies grappling to integrate modern data demands with outdated infrastructure.
Interoperability emerges as a critical concern, requiring standardisation of data formats and protocols to facilitate seamless data exchange across different vehicles, manufacturers, and systems. Bridging the skill gap is equally crucial, as the industry demands professionals proficient in data analytics, machine learning, and AI to navigate the complexities of automotive data effectively.
Real-time processing capabilities are essential for extracting insights from dynamic data sources like telematics and sensor data, as delays in analysis can jeopardise safety and performance. Moreover, the cost and infrastructure involved in implementing data-driven solutions pose a significant challenge, necessitating a careful balance between investment and returns. Lastly, regulatory compliance adds another layer of complexity, as automotive companies must navigate stringent data privacy regulations like GDPR while maximising the benefits of data utilisation.
While automotive data offers unprecedented opportunities for customisation, optimisation, and personalisation across the industry, addressing these challenges is imperative to unlock its full potential and ensure sustainable growth and innovation.
The Future of Automotive Data Adoption
In the future of automotive data adoption, several key trends are set to reshape the industry landscape. With the proliferation of connected cars, edge computing will emerge as a pivotal technology, enabling local processing of data within vehicles to reduce latency and enhance real-time decision-making, particularly as 5G networks facilitate faster communication between vehicles and edge servers. Predictive analytics will revolutionise maintenance practices and route optimisation, driven by historical data insights, thereby enhancing vehicle reliability and cost savings.
Connected services will deliver a seamless digital experience to drivers, offering personalised infotainment and over-the-air updates fueled by automotive data. This trend will also include features like remote diagnostics and real-time traffic information, further enhancing convenience. Data monetisation will unlock new revenue streams as companies explore innovative ways to leverage automotive data, from personalised insurance premiums to selling anonymised data to urban planners for traffic management.
However, with increased connectivity comes the pressing need for robust cybersecurity measures to safeguard data from breaches and ensure secure communication between vehicles. Supply chain optimisation will be driven by data analytics, streamlining processes from component sourcing to inventory management, leading to enhanced efficiency and reduced costs. Electric Vehicles (EVs) will generate unique data related to battery health and charging patterns, which will be crucial for optimising performance and addressing range anxiety.
Navigating regulatory compliance will be paramount, as stricter data privacy regulations shape how automotive data is collected, stored, and shared. In summary, the future of automotive data adoption promises a transformative shift towards more efficient, connected, and secure vehicles, powered by advanced analytics and technological innovations.
Merit’s Expertise in Data Aggregation & Harvesting for the Global Automotive Sector
Merit Data and Technology excels in aggregating and harvesting automotive data using AI, ML, and human expertise. Our capabilities include:
- Crafting end-to-end data pipelines and scalable data warehouses
- Designing compliant governance solutions for seamless integration
- Utilising high-volume, high-velocity data tools for nuanced insights
- Extracting retail product attributes and audience data
- Aggregating industry-specific data points for informed decision-making
Trusted by leading automotive brands, Merit drives innovation and efficiency by delivering refined, actionable insights.
Key Takeaways
Major Uses of Automotive Data:
- Predictive Maintenance: Forecast maintenance needs, extend vehicle lifespan.
- Improved Performance: Optimise design, enhance safety, fuel efficiency.
- Market Insights: Tailor products, understand consumer behavior.
- Supply Chain Efficiency: Reduce costs, enhance productivity.
- Electric Vehicle Integration: Inform decisions for successful EV adoption.
- Connected Cars: Enhance safety, enable personalised services.
- Competitive Advantage: Drive superior outcomes in various aspects.
Challenges & Gaps:
- Data Deluge: Manage influx, extract meaningful insights.
- Data Silos: Break barriers, foster collaboration.
- Data Quality: Ensure accuracy, avoid erroneous decisions.
- Privacy and Security: Balance utilisation, safeguard sensitive info.
- Legacy Systems: Integrate modern demands with outdated infrastructure.
- Interoperability: Standardise formats, facilitate seamless exchange.
- Skill Gap: Address need for proficient professionals.
- Real-time Processing: Ensure timely insights for safety and performance.
- Cost and Infrastructure: Balance investment for returns.
- Regulatory Compliance: Navigate stringent privacy regulations.
Future Trends:
- Edge Computing: Reduce latency, enhance real-time decision-making.
- Predictive Analytics: Enhance reliability, cost savings.
- Connected Services: Deliver personalised experiences.
- Data Monetisation: Explore new revenue streams.
- Cybersecurity Measures: Safeguard data, ensure secure communication.
- Supply Chain Optimisation: Streamline processes, reduce costs.
- Electric Vehicle Data: Optimise performance, address range anxiety.
- Regulatory Compliance: Navigate stricter privacy regulations.
Related Case Studies
-
01 /
A Hybrid Solution for Automotive Data Processing at Scale
Automotive products needed millions of price points and specification details to be tracked for a large range of vehicles.
-
02 /
A Digital Engineering Solution for High Volume Automotive Data Extraction
Automotive products required help to track millions of price points and specification details for a large range of vehicles.