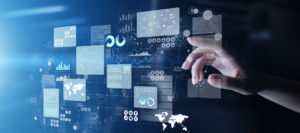
“Speed is one of the main benefits of real-time data processing; there is little delay between inputting data and getting a response,” says Splunk. “It also ensures that information is always current. Together, these features enable users to take accurately informed action in the minimum amount of time.”
It has never been easier for organisations to gather large quantities of data, nor to store it cheaply for the long term. As we have written elsewhere, “where once [an organisation] might only have had its own metrics to call on, today’s data-centric enterprises frequently supplement their own assets with second- and third-party data to produce a more nuanced and insightful result.”
However, Merit’s Senior Data Scientists say that “should that data reside on a server for too long without being acted upon, its value will decline. The rate at which data goes stale – and can no longer be considered ‘real-time’ – will differ between data types”. Aggregate sales figures for the car industry, used to drive investment decisions, might be considered real-time for a month or more. Polling data, in the run-up to an election, may lose its real-time value within an hour of a candidate making an unguarded remark or bungling a policy announcement.
Organisations must therefore gauge for themselves what constitutes ‘real-time’ when applied both to their own business model, and the data under discussion – and treat the latter as appropriate. Once they’ve done so, every additional metric gathered, which conforms to the real-time limit, can be used to enrich existing data sources to make more effective decisions.
Looking beyond immediate data points
Behavioural data can be some of the most enriching, yet hardest to gather and quickest to lose its ‘real-time’ value. You will often need direct contact with a subject or related entity to gather the kind of data on which you could build a recommendation engine or make predictions about future actions – yet it’s precisely this kind of real-time data enrichment that will give organisations an edge over competitors.
“Once you use behavioural data to optimise campaigns and improve KPIs, you’re well on your way to flexing your brand like a muscle that expands and contracts with your customer’s behaviour,” says James Phoenix.
Enriching real-time data with firmographics
But it’s not only real-time personal data that can be of value. As Integrate explains, “you can also append ‘firmographic’ information to company data. Firmographic data is to companies as demographic data is to people; it may include the company’s size, industry, structure, geographic location, revenue, and more.”
This firmographic data paints a broader, yet richer picture, effectively expanding the canvas to place your subject in context, helping you to target them more effectively in ongoing and future campaigns, and to better understand their role so you have a clearer idea of what their value to your own operation might be. Should they be replaced, this firmographic data can be applied to their replacement and, often, used to immediately enrich the data for other contacts within the same organisation. This immediate improvement of one metric on the basis of another ensures that both metrics qualify as real-time data, since the existing data has been verified and confirmed by the data point that has just been gathered.
As Snowplow describes, “when a user enters a company email into a form, and it just so happens that someone else from that same company interacted with that form earlier, data enrichment allows you to personalize content for that user based on the information you already gathered on that company”.
Real-time data integrated within a single platform
Naturally, care must be taken when integrating real-time data – particularly to ensure that it is not only timely, but accurate. Real-time data, such as stock prices, weather, and company news, is subject to change over time, and these changes must be reflected in the recorded data, with stale metrics removed when more relevant data becomes available.
When real-time data is integrated within a single platform, it is frequently cheaper and easier to maintain. You don’t have to ensure that updates are replicated across multiple locations, and contradictions are easier to spot. This dramatically simplifies the task of maintaining the single version of truth that is key to effective business development. The single version of truth ensures there is no contradiction in the ‘facts’ on which decisions are made across departments, which can occur when each is using data revised at different times.
Real-time data virtualisation
This is not to say that the data must all be stored in just one location, even though this makes a lot of sense; only that it should – ideally – be accessible through a single platform. There are many good reasons why an organisation may want to maintain multiple discrete databases, and move data between them. These include tracking past sales, for historical research, or to remain compliant with legal obligations, without having to keep stale data alongside current metrics.
However, there are equally good reasons why they might also want to expose only subsets of that data to users. Using data virtualisation, organisations can present business managers and other stakeholders with only that real-time information that is relevant to their role, without overloading them.
Yet, gathering real-time data in a single resource, like a data lake, pays dividends if the organisation wants to perform certain operations before making it available to users. It can even run background analytics and other operations while the data is still being gathered, and present the output, rather than the raw data, to those that need to use it. This helps end users to self-serve, while reducing the number of steps they need to take when analysing the content themselves in, say, a BI tool.
There is an additional, and especially important benefit to operating in this manner: by processing at least some of the data at the point of collection, before presenting it to users, that single view of the truth can be maintained. Stakeholders will be confident both that the data they are working with has been sanitised and is accurate, and that the speed at which it was analysed, whether using algorithms or AI, means it hasn’t lost its ‘real-time’ edge the way it may have done if that analysis was performed at the point of use.
Merit Group’s expertise in data virtualisation
At Merit Group, we work with some of the world’s leading B2B intelligence companies such as Wilmington, Dow Jones, Glenigan, and Haymarket. Our data and engineering teams work closely with our clients to build data products and business intelligence tools. Our work directly impacts business growth by helping our clients to identify high-growth opportunities.
Our specific services include high-volume data collection, data transformation using AI and ML, web watching, BI, and customised application development.
The Merit team also brings to the table deep expertise in building real-time data streaming and data processing applications. Our data engineering team brings to fore specific expertise in a wide range of data tools including Airflow, Kafka, Python, PostgreSQL, MongoDB, Apache Spark, Snowflake, Tableau, Redshift, Athena, Looker, and BigQuery.
If you’d like to learn more about our service offerings, please contact us here: https://www.meritdata-tech.com/contact-us
Related Case Studies
-
01 /
A Unified Data Management Platform for Processing Sports Deals
A global intelligence service provider was facing challenge with lack of a centralised data management system which led to duplication of data, increased effort and the risk of manual errors.
-
02 /
Bespoke Data Engineering Solution for High Volume Salesforce Data Migration
A global market leader in credit risk and ratings needed a data engineering solution for Salesforce data migration.